12 Powerful Ways AI is Revolutionizing Stock Market Trading Strategies in 2024
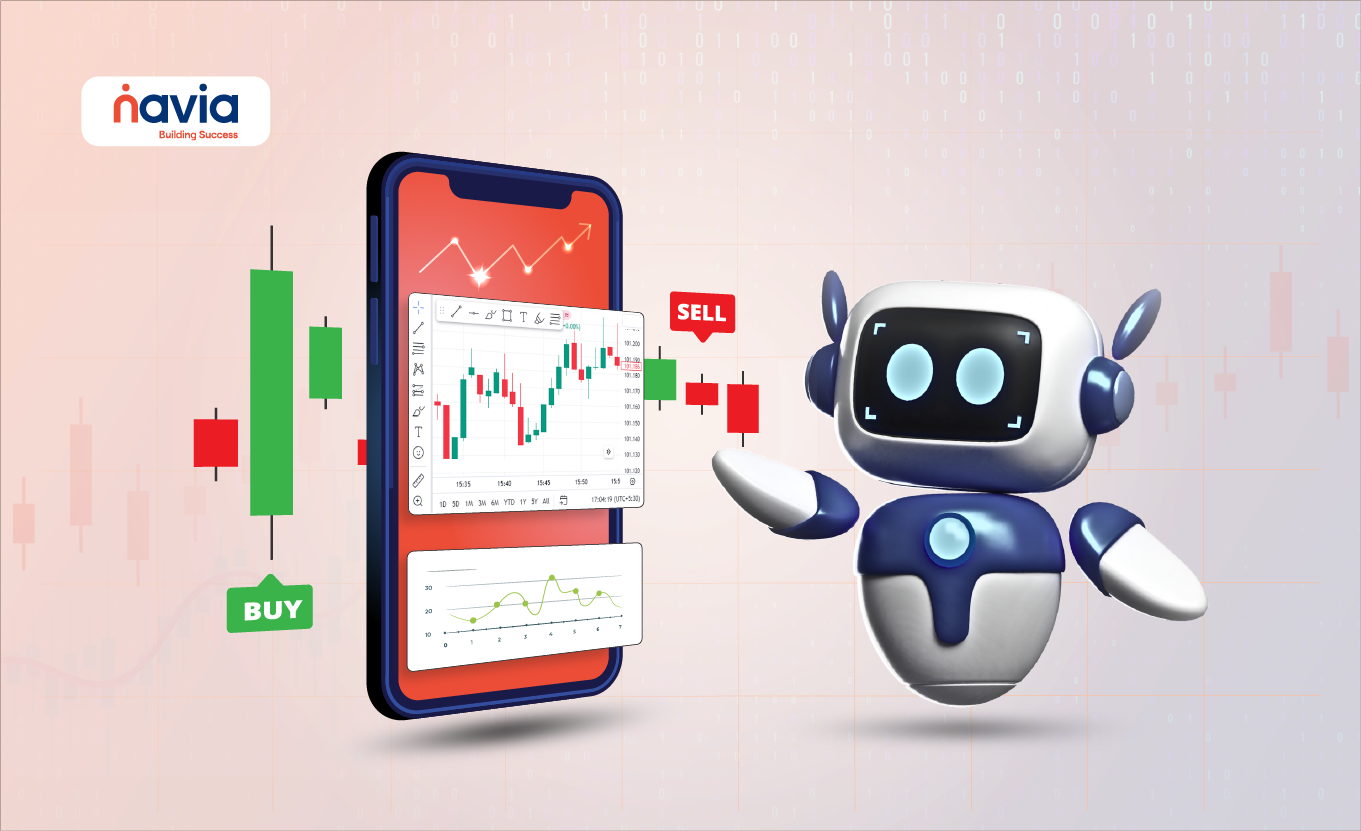
Table of Contents
Introduction
In 2024, AI is no longer just a buzzword in stock market trading—it’s a game-changer.
As AI algorithms become more sophisticated, they’re revolutionizing the way traders analyze markets, make decisions, and execute trades. From uncovering hidden patterns to predicting market moves, AI is helping traders gain a competitive edge like never before.
In this blog post, we’ll explore 12 ways AI is transforming stock market trading strategies in 2024:
⮞
How AI-powered sentiment analysis is unlocking real-time market insights
⮞
Why machine learning is the key to developing smarter trading algorithms
⮞
The role of predictive analytics in forecasting market moves and managing risk
⮞
How AI is enabling automated portfolio optimization for maximum returns
Whether you’re a seasoned trader or just starting out, understanding how AI is reshaping the stock market landscape is essential for success in 2024 and beyond. Let’s dive in.
AI-Powered Stock Market Analysis: Unlocking Profitable Insights
AI analyzes market sentiment and identifies hidden patterns for informed trading decisions
Machine learning algorithms predict stock prices and optimize portfolios
AI-driven insights give traders a competitive edge in fast-paced markets
Leveraging AI for Real-Time Market Sentiment Analysis
In today’s fast-paced stock market, staying on top of market sentiment is crucial for making informed trading decisions. AI algorithms have the power to analyze vast amounts of data from news articles, social media posts, and financial reports in real-time, providing traders with valuable insights into market mood and investor behavior.
By leveraging natural language processing (NLP) and machine learning techniques, AI can quickly identify positive or negative sentiment surrounding specific stocks, sectors, or the overall market. This real-time sentiment analysis enables traders to react swiftly to changing market conditions and adjust their strategies accordingly.
Case Study: Bloomberg’s AI-Powered Sentiment Analysis
Bloomberg, a leading financial data and media company, has developed an AI-powered sentiment analysis tool called “Bloomberg Sentiment Score.” This tool analyzes news articles and social media posts related to specific companies or industries, assigning a sentiment score ranging from -1 (most negative) to +1 (most positive). Traders can use these scores to gauge market sentiment and make informed decisions based on the prevailing mood.
Identifying Hidden Patterns and Correlations with AI
One of the key advantages of AI in stock market analysis is its ability to uncover complex relationships between various market factors that may not be apparent to human analysts. By analyzing vast amounts of historical data, including economic indicators, company fundamentals, and global events, AI algorithms can identify hidden patterns and correlations that can inform trading strategies.
For example, an AI system might discover a strong correlation between a company’s social media mentions and its stock price movements, or it may uncover a previously unknown relationship between a specific economic indicator and the performance of a particular sector. By leveraging these insights, traders can develop unique trading strategies and gain a competitive edge in the market.
⮞ Deep Learning for Pattern Recognition
Deep learning, a subset of machine learning, has proven particularly effective in identifying complex patterns in financial data. Convolutional Neural Networks (CNNs) and Recurrent Neural Networks (RNNs) are two types of deep learning architectures that have been successfully applied to stock market analysis. CNNs are well-suited for analyzing visual data, such as stock charts and technical indicators, while RNNs are designed to handle sequential data, such as time series of stock prices.
Predictive Analytics: Forecasting Stock Prices with AI
One of the most sought-after applications of AI in stock market trading is predictive analytics. By training machine learning models on historical price data, market trends, and other relevant factors, AI can generate predictions about future stock price movements.
While no prediction model is perfect, AI-driven forecasts can provide traders with valuable insights and help them make more informed decisions. Some popular machine learning algorithms used for stock price prediction include:
– Support Vector Machines (SVMs)
– Random Forests
– Gradient Boosting Machines (GBMs)
– Long Short-Term Memory (LSTM) networks
These algorithms can be trained on a variety of input features, such as technical indicators, fundamental data, and sentiment scores, to generate predictions over different time horizons, from short-term price movements to long-term trends.
AI-Driven Portfolio Optimization
In addition to predicting individual stock prices, AI can also be used to optimize entire investment portfolios. By analyzing the risk-return characteristics of various assets and considering factors such as diversification, correlation, and market conditions, AI algorithms can help traders construct portfolios that are well-suited to their investment goals and risk tolerance.
One popular approach to AI-driven portfolio optimization is the use of Genetic Algorithms (GAs). GAs are inspired by the principles of natural selection and evolution, and they work by iteratively “breeding” and selecting the best-performing portfolios from a large pool of candidates. By defining a fitness function that measures the desirability of a portfolio based on factors such as risk-adjusted returns, GAs can evolve towards increasingly optimal solutions over time.
Another approach is the use of Reinforcement Learning (RL), a type of machine learning that involves training an AI agent to make decisions in an environment with the goal of maximizing a reward signal. In the context of portfolio optimization, the AI agent can learn to make investment decisions based on market conditions and portfolio performance, adapting its strategy over time to maximize returns while managing risk.
The Future of AI in Stock Market Trading
As AI technologies continue to advance, it is clear that they will play an increasingly important role in stock market trading. While AI is not a silver bullet and cannot replace human judgment entirely, it can provide traders with valuable insights and help them make more informed decisions in a fast-paced, complex market environment.
Some potential future developments in AI-powered stock market analysis include:
➝ Improved sentiment analysis that incorporates a wider range of data sources, such as video and audio content
➝ More sophisticated pattern recognition and prediction models that can adapt to changing market conditions in real-time
➝ Increased integration of AI with other emerging technologies, such as blockchain and the Internet of Things (IoT), to provide even more comprehensive and reliable market insights
As the adoption of AI in stock market trading grows, it will be important for traders to stay informed about the latest developments and to carefully consider the potential benefits and risks of incorporating AI into their strategies. By leveraging the power of AI responsibly and in combination with human expertise, traders can unlock new opportunities for profitable insights and stay ahead of the curve in an ever-evolving market landscape.
Machine Learning for Trading Algorithms: Enhancing Efficiency and Accuracy
➝ Machine learning enables the development of sophisticated trading algorithms that process vast amounts of data and make rapid, accurate decisions
➝ Backtesting and optimization techniques help fine-tune trading strategies, ensuring they are well-suited to current market conditions and investor preferences
➝ Real-time adaptation allows trading algorithms to quickly respond to changing market dynamics, maximizing profitability and minimizing risk.
Developing sophisticated trading algorithms with machine learning
Machine learning has revolutionized the development of trading algorithms, enabling the creation of highly sophisticated systems that can process vast amounts of data and make rapid, accurate decisions. By leveraging the power of artificial intelligence, these algorithms can analyze historical market data, identify patterns and correlations, and generate predictive models that inform trading strategies.
One of the key advantages of machine learning-based trading algorithms is their ability to execute trades faster and more accurately than human traders. These algorithms can process and respond to market data in milliseconds, allowing them to capitalize on fleeting opportunities and minimize the impact of emotions and biases that can cloud human judgment. This increased efficiency and accuracy can lead to significant improvements in trading performance and profitability.
Moreover, machine learning algorithms can continuously learn and adapt to changing market conditions. By incorporating real-time data and feedback, these algorithms can refine their models and strategies over time, ensuring that they remain effective and relevant in the face of evolving market dynamics. This continual learning process allows trading algorithms to stay ahead of the curve and maintain a competitive edge in the fast-paced world of stock trading.
Case study: Renaissance Technologies
Renaissance Technologies, a renowned quantitative hedge fund, has been at the forefront of applying machine learning to trading algorithms. Their Medallion Fund, which employs complex machine learning models to identify profitable trading opportunities, has consistently outperformed the market, generating annualized returns of over 66% before fees between 1988 and 2018.
Backtesting and optimizing trading strategies using machine learning
Backtesting is a crucial step in the development of any trading strategy, as it allows traders to assess the performance and risk profile of their algorithms using historical data. Machine learning has greatly enhanced the backtesting process, enabling the rapid and thorough evaluation of trading strategies across a wide range of market scenarios.
By applying machine learning techniques to historical market data, traders can simulate the performance of their algorithms over different time periods and market conditions. This allows them to identify strengths and weaknesses in their strategies, as well as potential areas for improvement. Machine learning can also be used to optimize trading parameters, such as entry and exit points, position sizing, and risk management, to ensure that the algorithm is well-suited to current market conditions and investor preferences.
Robust backtesting and optimization are essential for developing trading strategies that can withstand the challenges of real-world market conditions. By leveraging machine learning to thoroughly test and refine their algorithms, traders can enter the market with greater confidence, knowing that their strategies have been rigorously validated and optimized for success.
⮞ Techniques for backtesting and optimization
Some common machine learning techniques used in backtesting and optimization include:
1. Genetic algorithms: These algorithms mimic the process of natural selection to iteratively optimize trading parameters, “breeding” successful strategies and discarding underperforming ones.
2. Reinforcement learning: This approach involves training an algorithm to make decisions based on rewards and punishments, allowing it to learn optimal trading strategies through trial and error.
3. Bayesian optimization: This technique uses Bayesian inference to efficiently explore the space of possible trading parameters, identifying optimal configurations with fewer iterations than traditional methods.
Real-time adaptation to changing market conditions
One of the most significant advantages of machine learning in trading is the ability of algorithms to adapt to changing market conditions in real-time. By continuously processing and analyzing incoming market data, these algorithms can identify shifts in market dynamics and adjust their strategies accordingly.
This real-time adaptation is made possible by the use of online learning techniques, which allow machine learning models to update their parameters and predictions as new data becomes available. By incorporating the latest market information into their decision-making process, trading algorithms can quickly respond to changing conditions, such as shifts in market sentiment, economic indicators, or geopolitical events.
The ability to adapt in real-time is crucial for maximizing profitability and minimizing risk in the fast-paced world of stock trading. By staying agile and responsive, machine learning-based trading algorithms can capitalize on emerging opportunities and mitigate potential losses, ensuring optimal performance in the face of ever-changing market conditions.
⮞ Reinforcement learning for real-time adaptation
Reinforcement learning is a particularly powerful technique for enabling real-time adaptation in trading algorithms. By framing the trading process as a series of decisions and rewards, reinforcement learning algorithms can learn to optimize their actions based on the feedback they receive from the market.
For example, a reinforcement learning-based trading algorithm might learn to adjust its position sizes or exit strategies based on the success or failure of its previous trades. By continuously learning from its experiences, the algorithm can refine its approach and adapt to changing market conditions, ensuring that it remains effective and profitable over time.
Predictive Analytics in Stock Trading: Forecasting Market Moves
AI-driven predictive models identify high-growth or undervalued stocks by analyzing vast datasets
➝ Predictive analytics helps traders anticipate market volatility and manage risk effectively
➝ AI algorithms can detect early warning signs of market instability or individual stock vulnerabilities
Harnessing the power of predictive analytics for stock selection
AI-driven predictive models have revolutionized the way traders approach stock selection. These sophisticated algorithms analyze vast datasets, including company financials, industry trends, and macroeconomic indicators, to identify stocks with high growth potential or undervalued assets.
By leveraging machine learning techniques like linear regression, decision trees, and neural networks, predictive analytics can uncover hidden patterns and correlations in historical data. This enables traders to make data-driven decisions based on a stock’s predicted future performance. For example, a model might identify a company with consistently growing revenue, a strong balance sheet, and a favorable industry outlook as a promising investment opportunity.
Enhancing risk management with predictive analytics
In addition to identifying promising investment opportunities, predictive analytics plays a crucial role in risk management. By analyzing historical data and real-time market trends, AI algorithms can anticipate market volatility and potential downturns. This allows traders to adjust their positions accordingly, minimizing losses and preserving capital during challenging market conditions.
Predictive models can also identify early warning signs of market instability or individual stock vulnerabilities. For instance, an algorithm might detect a sudden increase in a company’s debt-to-equity ratio, a decline in sales growth, or negative sentiment in news articles and social media posts. By flagging these potential risks early on, traders can make informed decisions to reduce their exposure or exit positions before significant losses occur.
Case study: Predictive analytics in action
One notable example of predictive analytics in stock trading is the success of Renaissance Technologies, a hedge fund known for its quantitative approach. The firm’s Medallion Fund, which heavily relies on AI and predictive models, has consistently outperformed the market, generating an average annual return of 66% from 1988 to 2018.
Predictive analytics and stock picking
While predictive analytics has undoubtedly enhanced traders’ ability to identify promising stocks, it’s essential to recognize that AI is not a silver bullet. Predictive models are only as good as the data they are trained on and the assumptions they make. As such, traders must exercise caution and combine AI-generated insights with fundamental analysis and human judgment.
That said, AI is becoming increasingly adept at stock picking. A study by Chong, Han, and Park (2017) found that a deep learning model outperformed traditional stock prediction methods, achieving an accuracy of 65.6% in predicting future stock prices. As AI continues to advance, its ability to identify high-potential stocks is likely to improve further.
The future of AI in stock trading
As AI technologies continue to evolve, their impact on the stock market will only grow. While it’s unlikely that AI will completely replace human traders in the near future, it will undoubtedly play an increasingly important role in decision-making processes.
For those looking to delve deeper into the topic of AI in stock trading, the book “Advances in Financial Machine Learning” by Marcos Lopez de Prado (2018) provides a comprehensive overview of the subject. The author explores various machine learning techniques and their applications in finance, offering valuable insights for traders and researchers alike. You can find more information about the book on [Amazon](https://www.amazon.com/Advances-Financial-Machine-Learning/dp/111946134X).
Automated Portfolio Optimization: Maximizing Returns and Managing Risk
➝ AI-driven portfolio optimization helps traders allocate assets effectively, manage risk, and maximize returns
➝ Automated portfolio rebalancing ensures the portfolio stays aligned with the trader’s risk tolerance and investment goals
➝ AI enables dynamic portfolio management, allowing traders to adapt to market changes and seize opportunities
Implementing AI-driven asset allocation strategies
AI algorithms have revolutionized the way traders allocate assets within their portfolios. By analyzing the risk-return profiles of various asset classes, AI can optimize portfolio allocation based on individual investor preferences. This means that traders can now easily create portfolios tailored to their specific risk tolerance and investment objectives.
One of the key benefits of AI-driven asset allocation is automated portfolio rebalancing. As market conditions change and asset prices fluctuate, AI algorithms can automatically adjust the portfolio’s holdings to maintain the desired risk-return balance. This ensures that the portfolio remains aligned with the trader’s goals, even in the face of market volatility.
🚩 The Impact of AI-driven asset allocation on portfolio performance compared to traditional methods.
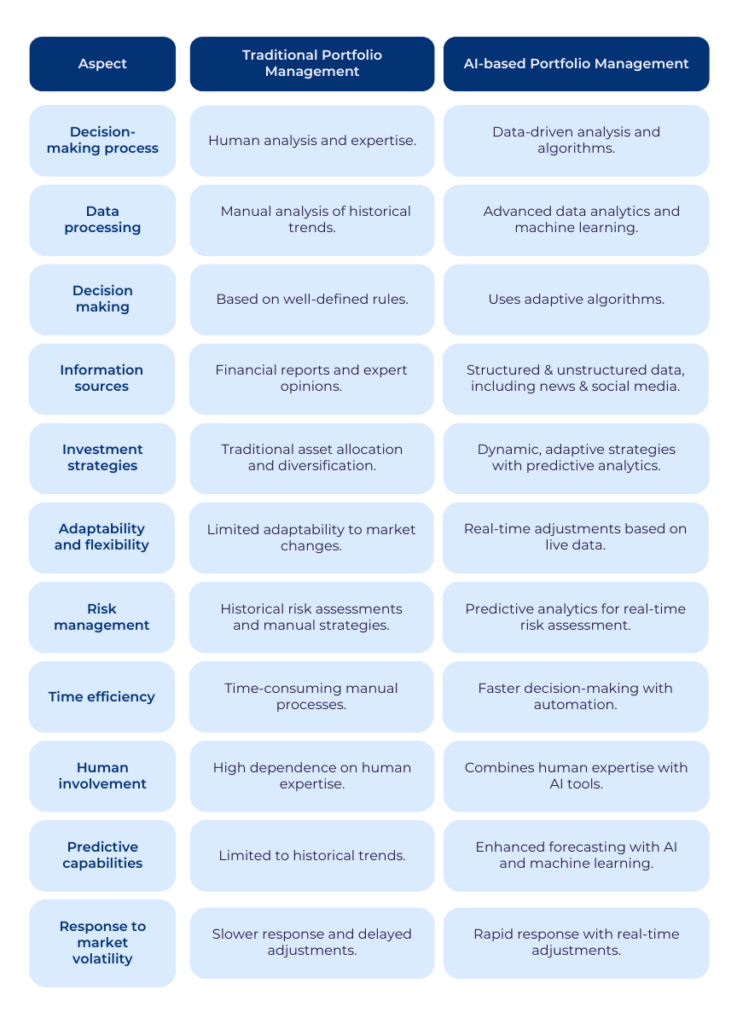
Case study: Wealthfront’s AI-powered asset allocation
Wealthfront, a leading robo-advisor, uses AI to create personalized investment portfolios for its clients. By gathering information about each investor’s risk tolerance, investment horizon, and financial goals, Wealthfront’s AI algorithms can construct portfolios that are optimized for their specific needs. This has resulted in strong performance for Wealthfront’s clients, with their portfolios outperforming the S&P 500 by an average of 1.8% annually from 2012 to 2021.
Leveraging AI for dynamic portfolio management
In addition to optimizing asset allocation, AI is also being used for dynamic portfolio management. This means that AI algorithms continuously monitor the portfolio and make adjustments in real-time based on changing market conditions and investor needs.
Dynamic portfolio management allows traders to quickly adapt to market shifts and capitalize on emerging opportunities. For example, if an AI algorithm detects a sudden increase in volatility in a particular sector, it can automatically reduce the portfolio’s exposure to that sector to mitigate risk. Similarly, if the algorithm identifies a promising investment opportunity, it can allocate funds accordingly to maximize potential returns.
Drawbacks of Heavy Reliance on AI for Dynamic Portfolio Management
● Over-optimization: AI fine-tuning portfolios based on historical data can lead to strategies that perform well in back testing but fail in real-world scenarios due to changing market conditions.
● Inability to account for unforeseen events: AI might struggle to account for unexpected events, such as political upheavals or natural disasters, which can cause sudden market shifts.
● Perpetuation and amplification of existing biases: AI systems can perpetuate and amplify biases present in the data they are trained on, leading to skewed investment strategies.
● Necessity of human oversight: Human oversight remains crucial to mitigate these risks and ensure a balanced, adaptable approach to portfolio management.
AI-driven risk management and portfolio stress testing
Another crucial aspect of portfolio optimization is risk management. AI can help traders identify and mitigate potential risks within their portfolios by conducting sophisticated stress tests and scenario analyses.
By simulating various market conditions and stress scenarios, AI algorithms can estimate how a portfolio would perform under different circumstances. This allows traders to identify potential weaknesses in their portfolios and make adjustments to improve resilience.
For example, an AI-driven stress test might reveal that a portfolio is overly exposed to interest rate risk. Armed with this knowledge, the trader can take steps to hedge against this risk, such as incorporating fixed-income securities with different maturities or using derivatives to offset potential losses.
Integrating AI with human expertise for optimal results
While AI has undoubtedly transformed portfolio optimization, it’s important to note that the most successful traders often combine AI-driven insights with human expertise. AI algorithms can process vast amounts of data and identify patterns that humans might miss, but they lack the contextual understanding and intuition that experienced traders bring to the table.
By integrating AI-driven portfolio optimization with human oversight and decision-making, traders can harness the best of both worlds. This hybrid approach allows for the speed and efficiency of AI while still incorporating the strategic thinking and adaptability of human traders.
Notable hedge funds and investment firms that have successfully integrated AI with human expertise for portfolio optimization:
🔸 Bridgewater Associates
● Overview: Bridgewater Associates, founded by Ray Dalio, is one of the largest and most successful hedge funds globally.
● AI Integration: The firm employs AI and machine learning to analyze vast amounts of economic data to identify market trends and develop trading strategies. Their “Pure Alpha” fund is known for its use of quantitative analysis combined with human insights to optimize investment decisions.
🔸 Two Sigma
● Overview: Two Sigma is a quantitative investment management firm founded by John Overdeck and David Siegel.
● AI Integration: Two Sigma heavily leverages AI, machine learning, and big data analytics in its trading strategies. They employ predictive modeling and data science techniques to uncover patterns and make data-driven investment decisions, supplemented by human expertise to fine-tune their models and strategies.
🔸 Renaissance Technologies
● Overview: Founded by Jim Simons, Renaissance Technologies is renowned for its Medallion Fund, which has achieved extraordinary returns.
● AI Integration: Renaissance Technologies utilizes sophisticated algorithms and statistical models to guide their investment strategies. They combine AI-driven quantitative analysis with the expertise of mathematicians and scientists to continually refine their trading models and identify profitable opportunities.
🔸 Man Group (AHL)
● Overview: Man Group is a global investment management firm, and AHL is its quantitative investment division.
● AI Integration: AHL integrates AI and machine learning in its investment process to analyze large datasets and identify trading signals. They blend AI models with human oversight to ensure robustness and adaptability in their strategies.
🔸 Point72 Asset Management
● Overview: Point72, led by Steve Cohen, is a leading asset management firm.
● AI Integration: The firm employs AI and machine learning techniques in its investment process to analyze data and identify investment opportunities. Human analysts and portfolio managers work alongside AI systems to enhance decision-making and optimize portfolios.
🔸 BlackRock
● Overview: BlackRock is one of the world’s largest asset management firms, with extensive experience in integrating technology into their investment processes.
● AI Integration: BlackRock uses AI and machine learning for risk management, portfolio optimization, and market analysis. Their Aladdin platform is a notable example, providing a comprehensive suite of tools for investment management that combines AI-driven insights with human expertise.
🔸 Goldman Sachs (Marcus)
● Overview: Goldman Sachs is a leading global investment bank and financial services firm.
● AI Integration: Through its consumer division, Marcus, Goldman Sachs utilizes AI to offer personalized financial advice and optimize investment portfolios. They combine AI algorithms with insights from their experienced analysts to provide tailored investment solutions.
The future of AI-driven portfolio optimization
As AI technology continues to advance, we can expect even more sophisticated and effective portfolio optimization strategies to emerge. Some potential developments on the horizon include:
➝ The integration of natural language processing (NLP) to analyze sentiment from news articles and social media, providing additional insights for portfolio optimization
➝ The use of reinforcement learning algorithms that can adapt and improve their strategies over time based on market feedback
➝ The incorporation of alternative data sources, such as satellite imagery and geo-location data, to identify investment opportunities and risks
By staying at the forefront of these developments, traders can continue to refine their AI-driven portfolio optimization strategies and maintain a competitive edge in the fast-paced world of stock market trading.
The Widespread Adoption of AI in Stock Trading
➝ AI-powered trading systems are being rapidly adopted by financial institutions and individual traders alike
➝ The share of stock trading volume attributed to AI algorithms is steadily increasing year over year
➝ As AI advances, its impact on stock market trading is expected to grow exponentially
The growing prevalence of AI-driven trading in financial markets
The adoption of AI in stock trading has been on a steep upward trajectory in recent years. Institutional investors, hedge funds, and even individual traders are increasingly turning to AI-powered trading systems to gain a competitive edge in the market. These sophisticated algorithms can process vast amounts of data at lightning speeds, identifying patterns and generating insights that would be impossible for human traders to discern.
According to a report by JPMorgan, around 60% of trading in the US stock market is now done by machines, many of which rely on AI and machine learning algorithms. This trend is not limited to the US; globally, the share of stock trading volume attributed to AI algorithms has been steadily rising. In 2020, AI-driven trading accounted for an estimated 80% of total trading volume in developed markets.
The widespread adoption of AI in stock trading can be attributed to several factors. First and foremost, AI algorithms can process and analyze massive amounts of data much faster than human traders. This allows them to identify trends, patterns, and opportunities in real-time, enabling faster and more accurate decision-making. Additionally, AI-powered trading systems can operate 24/7, continuously monitoring the markets and executing trades without the need for human intervention.
The future of AI in stock market trading
As AI technologies continue to advance at a rapid pace, their impact on stock market trading is expected to grow exponentially. The integration of AI with other emerging technologies, such as blockchain and quantum computing, may further revolutionize trading strategies and open up new possibilities for investors.
One area where AI is poised to make significant strides is in the realm of predictive analytics. By analyzing vast amounts of historical data and real-time market information, AI algorithms can potentially predict future market movements with greater accuracy than ever before. This could lead to the development of more sophisticated trading strategies that can adapt to changing market conditions in real-time.
However, it is important to note that while AI is transforming the landscape of stock trading, human expertise and oversight remain crucial in developing and monitoring AI-driven trading systems. The most successful trading firms will likely be those that can effectively combine the power of AI with the intuition and experience of seasoned traders.
⮞ The role of human expertise in AI-driven trading
While AI algorithms can process and analyze data at speeds and scales that are impossible for human traders, they still rely on human expertise for their development and oversight. Experienced traders and financial professionals play a critical role in designing, testing, and refining AI trading strategies to ensure they are effective and aligned with the firm’s overall investment objectives.
Moreover, AI-driven trading systems are not infallible. They can be susceptible to biases, errors, and unexpected market events that may require human intervention to correct. As such, the most successful trading firms will likely be those that can strike a balance between leveraging the power of AI and maintaining a strong human element in their trading operations.
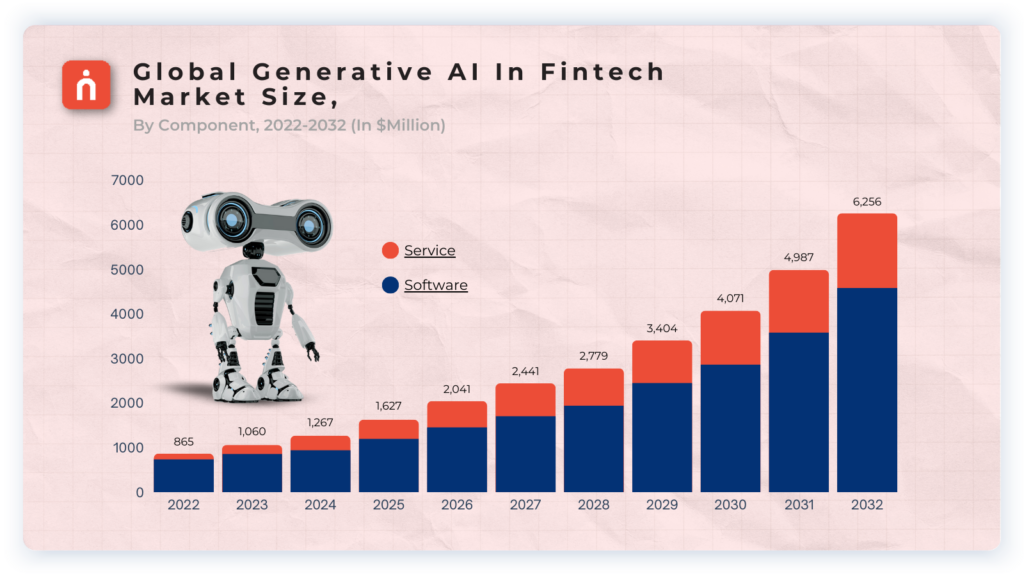
Source: How to Integrate AI into a Stock Trading App (vlinkinfo.com)
As the adoption of AI in stock trading continues to accelerate, it is clear that this technology is revolutionizing the way investors approach the market. By harnessing the power of machine learning and predictive analytics, traders can gain a competitive edge and potentially generate higher returns. However, it is important to remember that AI is not a panacea; human expertise and oversight remain essential components of successful trading strategies in the age of artificial intelligence.
Understanding the Fundamentals of AI in Stock Trading
➝ AI in stock trading involves using machine learning and natural language processing to analyze market data and make informed investment decisions
➝ AI algorithms can process vast amounts of data, identify patterns, and predict market movements faster and more accurately than human traders
➝ While AI has the potential to revolutionize stock trading, it is not a perfect solution and requires human oversight to address regulatory and ethical concerns
What is AI in the context of stock trading?
AI, or artificial intelligence, refers to the application of machine learning, natural language processing, and other computational techniques to analyze market data and make trading decisions. In the context of stock trading, AI algorithms can process and interpret vast amounts of structured and unstructured data, including financial statements, news articles, and social media sentiment.
The primary goal of AI in stock trading is to identify patterns, predict market movements, and optimize investment strategies. By leveraging the power of AI, traders can gain valuable insights into market trends, risk factors, and potential opportunities that may not be apparent to the human eye.
⮞ Machine learning in stock trading
Machine learning, a subset of AI, plays a crucial role in stock trading. Machine learning algorithms can be trained on historical market data to identify patterns and relationships between various factors, such as price movements, trading volume, and economic indicators. These algorithms can then use this knowledge to make predictions about future market behavior and generate trading signals.
For example, algorithms like random forests, support vector machines, and recurrent neural networks are commonly used in stock trading to analyze data and make predictions.
The benefits of AI for stock market traders
One of the primary benefits of AI in stock trading is its ability to enable traders to make data-driven decisions based on real-time market insights and predictive analytics. By analyzing vast amounts of data and identifying patterns that may not be apparent to human traders, AI algorithms can help traders make more informed investment decisions.
Moreover, AI algorithms can execute trades faster and more accurately than human traders, reducing the impact of emotions and biases on trading decisions. This is particularly important in fast-paced, high-frequency trading environments where split-second decisions can make a significant difference in returns.
AI-driven trading strategies can also help traders optimize returns, manage risk, and adapt to changing market conditions. By continuously learning from new data and adjusting trading strategies accordingly, AI algorithms can help traders stay ahead of the curve and capitalize on emerging opportunities.
Addressing concerns and limitations of AI in stock trading
While AI has the potential to revolutionize stock trading, it is not a perfect solution and comes with its own set of concerns and limitations. One of the primary concerns surrounding AI in stock trading is the potential for biased or flawed decision-making.
AI algorithms are only as good as the data they are trained on, and biased or incomplete data can lead to flawed trading decisions. For example, if an AI algorithm is trained on historical data that does not accurately represent the current market conditions, it may generate trading signals that are no longer relevant or effective.
Another concern is the lack of transparency and interpretability of some AI algorithms, particularly deep learning models. These “black box” models can make it difficult for traders to understand how the algorithm arrived at a particular decision, which can be problematic from a regulatory and accountability perspective.
The global AI trading market was valued at $18.2 billion in 2023 and is expected to nearly triple in size by 2033. This growth highlights the need for regulatory oversight to ensure fair and transparent markets.
⮞ The importance of human oversight
Despite the concerns and limitations of AI in stock trading, it is important to note that AI is not meant to replace human traders entirely. Instead, AI should be viewed as a tool to augment and enhance human decision-making.
Human oversight and judgment remain critical in ensuring that AI algorithms are used responsibly and ethically in stock trading. Traders must be able to interpret and validate the outputs of AI algorithms, and make informed decisions based on a combination of data-driven insights and their own expertise and intuition.
For further reading on the role of human oversight in AI-driven trading, consider “The Man Who Solved the Market” by Gregory Zuckerman or “Advances in Financial Machine Learning” by Marcos Lopez de Prado.
Getting Started with AI-Driven Stock Trading
➝ Developing a solid understanding of AI concepts and techniques is crucial for traders looking to leverage these tools effectively
➝ Researching and comparing various AI-driven trading platforms can help traders find the most suitable options for their needs
➝ Gradually incorporating AI-driven insights into existing trading strategies is essential for long-term success and risk management
Educating yourself about AI and its applications in stock trading
Before diving into AI-driven stock trading, it’s essential for traders to develop a solid foundation in AI concepts, techniques, and their potential impact on financial markets. This knowledge will help traders make informed decisions when selecting and implementing AI tools in their trading strategies.
To stay up-to-date with the latest developments in AI and financial technology, traders should regularly engage with relevant content, such as industry news, research papers, and expert blogs. This ongoing learning process will enable traders to identify new opportunities and adapt their strategies as AI technology evolves.

Attending workshops and conferences
Attending workshops, webinars, and conferences focused on AI in finance can provide valuable insights and networking opportunities. These events often feature industry experts sharing their experiences, best practices, and predictions for the future of AI-driven trading. By participating in these events, traders can gain a deeper understanding of how AI is being applied in real-world trading scenarios and connect with like-minded professionals.
Identifying reliable AI-powered trading tools and platforms
With the growing popularity of AI in stock trading, numerous tools and platforms have emerged in the market. To find the most suitable options for their needs, traders should thoroughly research and compare various AI-driven trading solutions.
When evaluating AI-powered trading tools and platforms, traders should consider factors such as:
1. Track record: Look for platforms with a proven history of successful trades and positive user feedback.
2. Transparency: Choose providers that are transparent about their AI algorithms, data sources, and performance metrics.
3. User reviews: Read reviews from other traders to gauge the platform’s reliability, user experience, and customer support.
4. Compatibility: Ensure that the AI tools integrate seamlessly with your existing trading setup and strategies.
Additionally, it is crucial to select reputable providers that prioritize data security, regulatory compliance, and customer support. This will help traders safeguard their investments and access timely assistance when needed.
Integrating AI into your existing trading strategy
When incorporating AI into your trading approach, it’s recommended to start gradually rather than relying solely on AI-driven decisions. This allows traders to assess the performance of AI tools and make necessary adjustments before fully committing to them.
To begin, traders can test AI-powered trading algorithms on historical data and in demo accounts. This enables them to evaluate the algorithms’ effectiveness without risking real capital. By analyzing the results, traders can identify strengths, weaknesses, and areas for improvement in their AI-driven strategies.
⮞ Monitoring and evaluating AI performance
As traders integrate AI into their trading strategies, it’s essential to regularly monitor and evaluate the impact of AI on trading outcomes. This involves tracking key performance indicators (KPIs) such as return on investment (ROI), win rate, and risk-adjusted returns. By comparing these metrics against benchmark indexes and personal trading goals, traders can determine whether their AI-driven strategies are delivering the desired results.
🚩 The performance of AI-driven trading strategies over time:
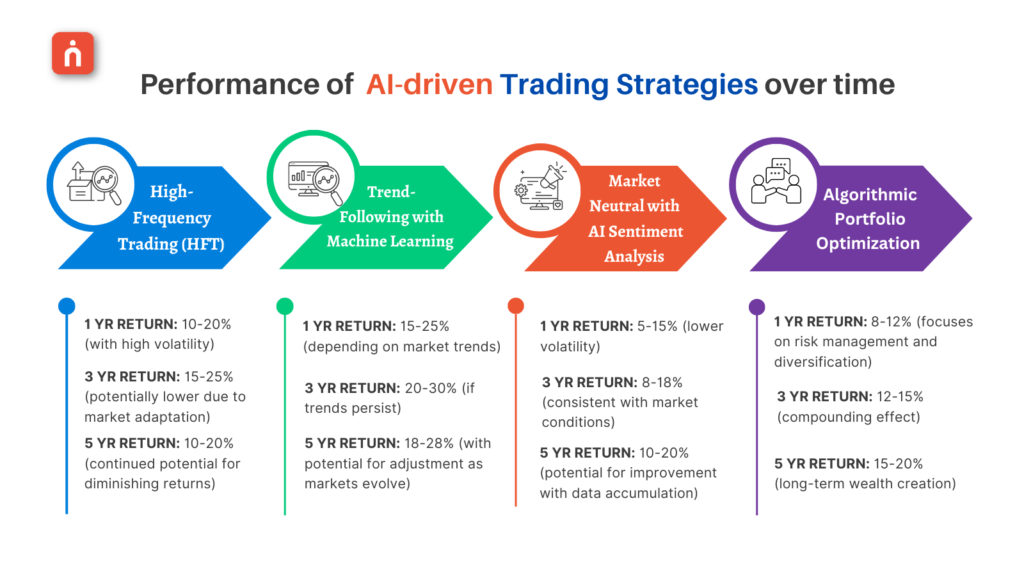
Continuously monitoring and refining AI-driven trading strategies based on performance data is crucial for long-term success and effective risk management. As AI technology advances and market conditions evolve, traders must remain adaptable and willing to make necessary adjustments to their AI-powered trading approaches.
AI: Your Trusted Ally in the Stock Market
AI is transforming the way traders approach the stock market, offering powerful tools and insights to navigate the complexities of investing. By harnessing the potential of AI-driven analysis, predictive analytics, and automated portfolio optimization, traders can make more informed decisions, manage risk effectively, and adapt to the ever-changing market landscape.
As AI continues to revolutionize stock trading, it’s essential to stay informed and embrace these cutting-edge technologies. Educate yourself about AI’s applications in finance, explore reliable AI-powered trading platforms, and gradually integrate these tools into your existing strategy. Remember, while AI is a valuable ally, human expertise and oversight remain crucial in developing and monitoring trading systems.
Are you ready to embark on your AI-driven trading journey? Start by assessing your current trading approach and identifying areas where AI could enhance your decision-making process. With the right knowledge, tools, and mindset, you can harness the power of AI to achieve your investment goals and thrive in the stock market of the future.
We’d Love to Hear from you